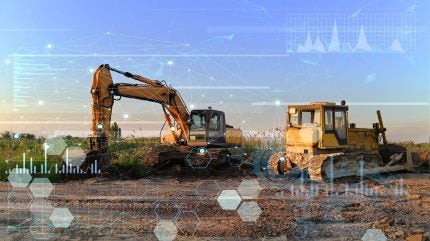
The mining industry is facing mounting productivity pressure, responding to ramping demand for clean energy minerals and supply chain diversification, and ongoing geopolitical tensions. In response, operators are fast tracking digital tools to help boost operational efficiencies and transparency, with AI emerging as a crucial tool in this new digital landscape.
One area AI is helping to reshape is asset maintenance, allowing companies to track and monitor the performance of assets throughout a mine site and transform operations from reactive to predictive models. With the rise of Industry 4.0 and sensor technology, predictive tools are getting smarter and sleeker, collecting larger amounts of data on mining assets to provide real-time insight into operations – and allowing companies to intervene before a problem occurs.
We look at how AI is changing predictive maintenance capabilities, and what the future holds for the tech in mining and beyond.
The dangers of poor mine maintenance
Traditionally, miners have been reliant on preventative maintenance models, meaning they intervene once a problem has already occurred, or they rely on an assumed maintenance schedule based on usage patterns.
According to Michael Zolotov, CTO of industrial tech company Razor Labs, before the rise of AI even predictive maintenance models had severe limitations, relying on manual analysis of an asset.
“These simplistic, threshold-based systems can generate numerous false alarms, causing unnecessary maintenance actions or ignoring critical issues,” explains Zolotov. “Because classic condition monitoring (vibration, oil, thermography) is manual, by definition it cannot be done continuously. It is usually performed every few months and therefore it misses faults, especially those that deteriorate quickly.”
How well do you really know your competitors?
Access the most comprehensive Company Profiles on the market, powered by GlobalData. Save hours of research. Gain competitive edge.
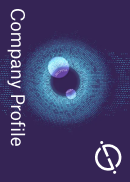
Thank you!
Your download email will arrive shortly
Not ready to buy yet? Download a free sample
We are confident about the unique quality of our Company Profiles. However, we want you to make the most beneficial decision for your business, so we offer a free sample that you can download by submitting the below form
By GlobalDataMaintaining an effective predictive maintenance schedule is more instrumental to a site’s operation than may initially be apparent. A 2022 report from Deloitte found poor maintenance strategies can reduce a plant’s productivity by between 5% and 20% – costing industrial manufacturers an estimated $50bn each year.
AI is stepping in to mitigate these issues, and investment into the tech is already booming. A GlobalData report estimated the AI industry will reach $908.7bn by 2030, growing by 35.2% between 2022 and 2030. Additionally, 40% of miners surveyed believe AI will be used to bolster predictive maintenance capabilities.
The rise of AI in predictive maintenance
The use of AI in predictive maintenance has been steadily rising over the past decade, coinciding with advancements in AI, the Industrial Internet of Things (IIoT) and Industry 4.0, says Zolotov.
The impact of these new tools on predictive maintenance includes advanced analytics capabilities, improved decision making and increased efficiency. Perhaps the most significant driver of AI’s adoption, however, is its ability to collect, store and analyse greater volumes of data than ever before.
Glenn Kerkhoff, industry principal of mining, metals and minerals at industrial software company Aveva, says AI has already had a “tremendous” impact on the mining sector, informing the development of smart factory tools such as cloud computing and digital twins.
“On Industry 4.0 – which is quickly becoming industry 5.0 – technologies such as cloud computing are enabling real-time collaboration on data across multiple operations, enabling a single repository for data, analytics and visualisation,” Kerkhoff says. “This ensures mining companies have up-to-date access to all data at the same time, regardless of location or remoteness of sites.”
The vast amounts of data now available to companies also provides them with a more detailed foundation to create accurate digital twins of assets – virtual copies of physical equipment that can be used to test and monitor operational processes.
“Digital twin technology allows organisations to test, modify and plan better than ever before,” Kerkhoff adds. “The emergence of predictive and AI modelling enhances the capability of the digital twin by modelling scenarios and processes, allowing organisations to test capabilities well in advance of physical world deployment.”
The value of data
Currently, miners could be wasting huge amounts of data because they don’t know how to use it. Forrester estimates 60–73% of data in an organisation is never successfully used for any strategic purpose.
With AI – specifically, machine learning – new predictive maintenance models are capable of scanning thousands of stored data points to identify issues and trends weeks, or even months, before they occur.
“Mining technology already has real-time monitoring sensors and data gathering capabilities, but the data is at present not being fully utilised,” according to GlobalData’s AI report.
“Machine learning can process and understand this data in real time, using it to predict when a machine is about to fail… This will eradicate the catastrophic machine failures in the mines, while also reducing repair costs for the mining company,” the report states.
Rolling out predictive analytics in the mining industry
Savings from data-driven predictive maintenance models are already being seen across the mining industry.
One example is Votorantim Cimentos, Brazil’s largest cement manufacturer, which is working alongside Aveva to roll out a predictive analytics solution across six of its mining sites – resulting in savings of $5.5m in corrective maintenance costs.
Aveva has also been working with Rio Tinto, using predictive maintenance to monitor assets across its North American operations including hydro-electric turbines in its Canadian aluminium operations.
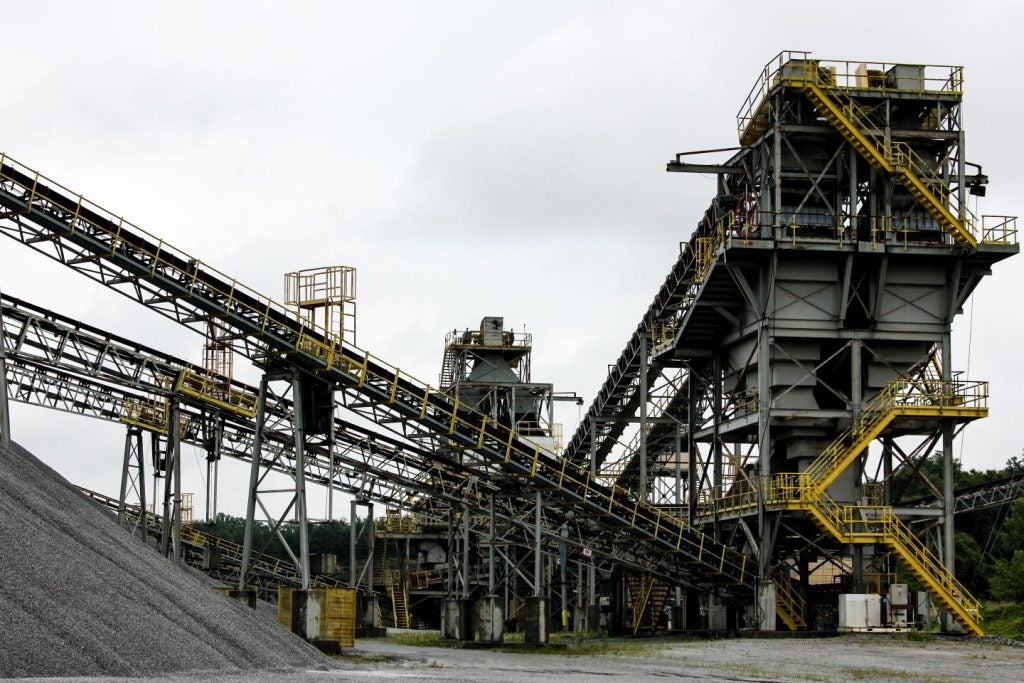
“Rio Tinto… relies on a data infrastructure that provides real-time insight across multi-site operations,” Kerkhoff explains. “Our PI System feeds data in real time to our predictive maintenance application, which in turn uses AI and advanced analytical algorithms to monitor asset health over a range of different asset classes,” he adds.
“Connecting design and engineering tools allows Rio Tinto to streamline project delivery, making new project start-up and ongoing operations more efficient.”
AI implementation challenges still facing the sector
Digital tools are more accessible than ever before; both in terms of cost and the skills required to use them. However, obstacles remain to the universal roll out of AI-enabled tools across mine operations.
According to GlobalData’s report into AI’s adoption, the cost of technology and the need for the tech to be proven were identified by respondents as primary barriers, with roughly 50% and 45% of respondents, respectively, identifying these as concerns.
Despite these hesitations, the use of AI is only expected to continue increasing as the industry seeks sustainable, efficient operations.
According to Zolotov, beyond predictive maintenance AI could see significant changes in autonomous operation, enhanced exploration and environmental management.
“The future of AI in mining is promising, with the potential to further transform the industry in several ways,” Zolotov says. “AI can enable more autonomous mining operations, analyse geological data more effectively and optimise the entire supply chain.”
Is it time for more miners to invest in predictive maintenance?
Indeed, while the full potential of AI – in predictive maintenance and beyond – is yet to be seen, companies unwilling to take a leap of faith with its deployment may find themselves left behind.
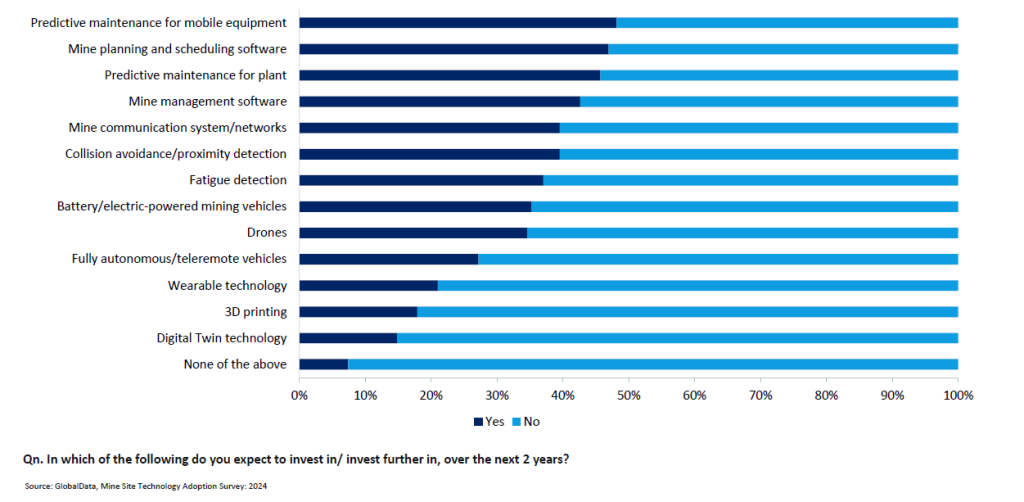
“As mines transition to decarbonised operations, AI will be critical,” Kerkhoff notes.
“AI’s ability to summarise, analyse, make sense of data quickly, and predict outcomes will ensure mining companies can determine the ideal process required for a given task to avoid incidents before they occur, as well as help companies reduce energy consumption.”
“On the profitability side, what is better for the environment is also better for the business, and reducing inefficiencies can boost the bottom line and assist mining companies in meeting their stated climate targets. AI is key, as it reduces human error, speeds up the time for analysis and ensures companies don’t miss opportunities to become more efficient and more sustainable,” he concludes.