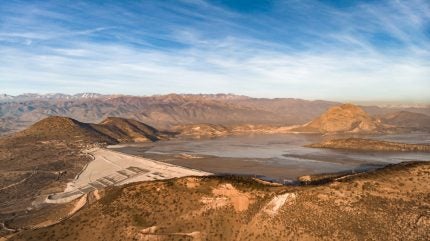
The need for better risk management and early-warning systems for tailings dam management is an increasingly important part of mining companies’ operations. The issue was the focus of renewed attention after the Brumadinho dam collapse in Brazil in 2019.
In response to the disaster, the 2020 Global Tailings Review released a new Global Industry Standard on Tailings Management, emphasising the need for appropriate monitoring of tailings facilities throughout their life cycle.
While this framework is more a guideline than a legal requirement, it indicates where the industry is heading, and is expected to be used as the basis for global standards that are set to be introduced in the coming years.
In this context, rapidly evolving digital tools like AI and machine learning (ML) are better placed than ever to help mining companies meet updated compliance standards, supporting tailings management and mitigating tailings dam failures. Looking ahead, the tech could also play a crucial role in taking mitigation a step further by helping companies recycle their waste products, reducing tailings altogether and improving mines’ circularity in the process.
AI as a monitoring tool
A key element of the proposed tailings standards is the implementation of robust monitoring systems to prepare for emergency responses, with the goal of achieving “zero harm to people and the environment”.
To achieve this, mining companies are increasingly deploying Internet of Things (IoT) sensors throughout tailings storage facilities, gathering information on factors such as soil moisture, temperature, rainfall and seismic shifts; all of which can provide early warnings of dam collapse.
A key issue AI and machine learning can help with here is in the consolidation and analysis of the vast amounts of data gathered from these sensors – data that is only set to continue growing alongside the number of tailings facilities.
“We are now seeing almost all of the major players doing this,” says Rebecca Kahrhoff, industry manager for mining at geographic information system (GIS) software company Esri. “They are linking IoT sensors out in the field to create a real-time map that is viewable both in remote locations and in an office.”
“The beauty of it is, you are not only using this for real-time monitoring,” she added. “Once the present becomes the past, then you have a historical catalogue. You have a centralised, single source of truth for that data that can be incredibly valuable for tracking change.”
Examples can be seen in artificial intelligence of things (AIoT) company VROC’s AI platform that analyses mine and weather data to provide predictions on elements such as tailings dam water levels, drainage, structural integrity and stability, dust and seismic activity.
Similarly, AI software company SAP is working alongside organisations such as EY to create a ‘health-status monitor’, consolidating data from cameras, sensors, satellites and drones and using predictive algorithms to monitor for issues, and trigger maintenance and repair requests.
“AI has the potential to connect data in a better way than an individual can do, looking at various data sources and having to connect the dots in his head,” says Ruediger Schroedter, global lead for mining at SAP. “AI can be useful in identifying areas where changes have happened, to read water levels, and used in simulations to predict where flood waves might go to improve evacuation plans.”
The future of AI for tailings
While its full potential in tailings management still unfolding, there are other avenues where AI is already being tipped to offer significant benefits – including the task of recycling waste materials to generate more marketable products.
Extracting these materials is not currently economically viable, Schroedter says. However, the scope for AI to improve mines’ circular processes and reduce tailings altogether is a potentially huge market.
“There is a lot of untapped potential in mine waste, and AI-powered geospatial tools can help identify opportunities and streamline the process,” says Kahrhoff. “A few ways AI is making an impact include using AI-driven remote sensing (via satellites and drones) to detect valuable minerals still present in tailings, while machine learning models analyse geochemical data to pinpoint the best sites for reprocessing.
“GIS-integrated IoT sensors can also be used to optimise recovery, tracking moisture levels, particle size and mineral concentrations in real time, and feeding this data into AI models to help refine separation techniques like flotation and leaching,” she adds.
While projects such as these remain in their early stages, moves towards achieving this are already under way, with Brazilian miner Vale announcing last year that it aims to generate 10% of its iron ore from reused tailings.
In addition, the Australian Mineral Industry Research Association has a proposed a project using ML to identify ways of repurposing tailings. The proposal contains two solutions: the first using AI to separate waste and the second using 3D modelling to determine the valuable materials within tailings.
Yet despite the significant benefits AI could bring to a mine’s sustainability and safety, as with any new tech its uptake faces challenges in integration and scalability.
Obstacles to uptake of AI in tailings management
According to Kahrhoff, the first (and potentially one of the greatest) challenges mining companies must face is in the realm of data collection.
As most sensor systems operate independently, creating a holistic platform to collect and showcase this data is a common but essential challenge to overcome.
“You have to decide what data is important to you, how you want it structured, and then you have to build out that structure,” Kahrhoff says. “It can be a cumbersome mission; it is really the first hurdle, especially if you are moving from paper to digital.”
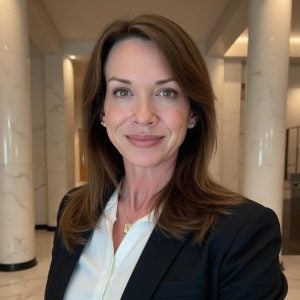
Another issue typical of any relatively new digital tool is adoption reluctance among workers, many of whom may be unfamiliar with the technology and therefore hesitant to trust it. Particularly in an industry such as mining, which has a lot of generational employees and passed-down knowledge, getting workers to abandon long-held practices in favour of new methods can be a significant challenge.
The solution, Kahrhoff suggests, lies in the tools’ usability.
“You have to make the tools user-intuitive,” she says. “Get people comfortable using them and they will adopt them.”
Similarly, Schroedter believes digital tools’ effectiveness need to be proven before widespread uptake can be expected and, as the technology is still relatively new in the field, real-world examples do not yet have the longevity needed to showcase tangible benefits.
“Track records of effectiveness and reliability or being better than traditional approaches are not there yet,” he says. “That may slow adoption as mining companies are risk averse, especially in this area.”
Importantly, none of these challenges are seen as insurmountable in AI’s adoption (in tailings management and beyond), and the consensus seems to be that mining companies unwilling to take the leap will be left behind.
Indeed, with AI still rapidly developing, there is a sense that mining companies are only at the beginning of their AI journeys.
“We have seen AI and ML facilitating rapid response in emergency situations, and we have seen it being used for satellite imagery capture and weather prediction,” says Kahrhoff. “The truth is, we are really just at the beginning of its use. It will probably revolutionise all tech, but we don’t yet know exactly where it is going.”